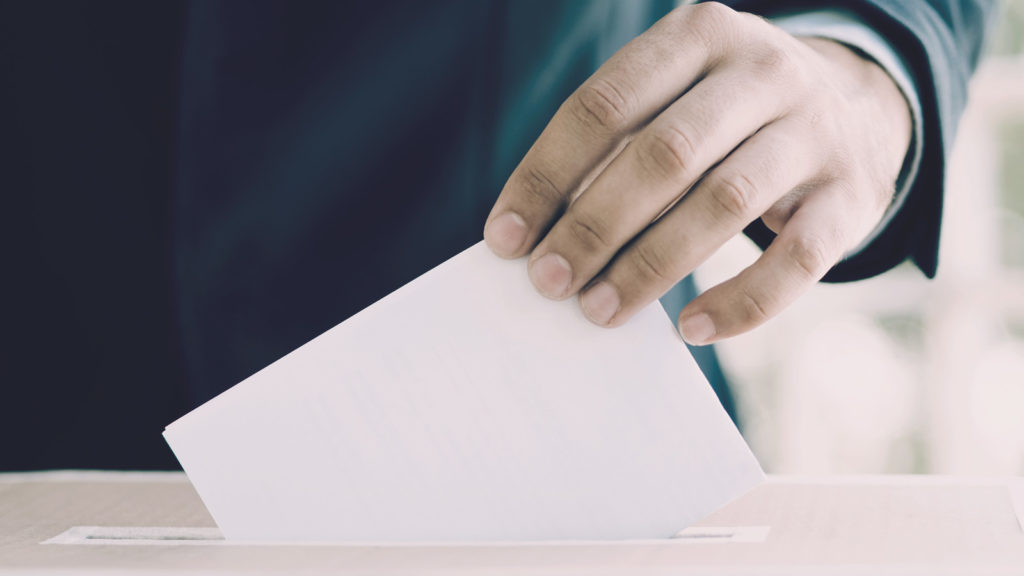
At a glance
The 2016 Brexit referendum changed the face of politics in the UK. In the aftermath, it was clear to us that a different approach to political research was needed to better understand who people are voting for and why.
We took this opportunity to take a fresh look at how voting intention is measured. We applied data science to demographic data to identify the key battlegrounds, and the decisive issues that had the biggest influence on a person’s vote.
On election day 2019, our analysis correctly identified 55 of the 75 seats that changed hands –more than any other modelled prediction.
The full story
Our analysis of the 2016 EU referendum results highlighted that rather than the traditional approach of focusing on the most marginal seats, the 2019 general election predictions should focus on the values shaping voting behaviour. A deeper dive into the data enabled us to identify our five values-based battlegrounds, covering 192 constituencies.
A specifically designed sample study of 8,500 people – consisting of around 1,000 people in each of the five battlegrounds and a 3,000 nat rep control group – allowed us to measure the trade-offs voters were weighing up when deciding who to vote for.
Were they more worried about the prospect of Brexit or the risk of Jeremy Corbyn being Prime Minister? Did they place more importance on the NHS than Brexit?
Further number crunching identified which trade-offs were most important in each battleground, from which the probability of each party winning each constituency in that area could be calculated.
The key to any general election is in identifying which constituencies will change hands. In the 2019 general election, 75 constituencies were won by a different party than at the previous general election.